With flow cytometry, as with many technologies that enjoy continuous use, we can see an evolution of capabilities. Spectral analysis, image capture, and artificial intelligence are just a few of the capabilities that are being added to flow cytometry systems. Overall, these capabilities are meant to help flow cytometry users examine smaller, rarer, and more complex targets. Some of the targets coming into view are DNA clusters (useful for detecting mutations), extracellular vesicles, and cells from tiny subpopulations. Also, increasingly capable flow cytometry systems are getting better at revealing the diversity of cell populations.
Spectral flow
“In polychromatic flow cytometry, you have one detector for one dye,” says Purdue University’s Paul Robinson PhD, “but in spectral flow cytometry, that is not the case.” Whereas polychromatic flow cytometry involves measurements of signal intensity, spectral flow cytometry evaluates spectral signatures. For each cell, there is a unique spectral signature. It is created by both the cell’s fluorochrome markers and the cell’s natural autofluorescence.
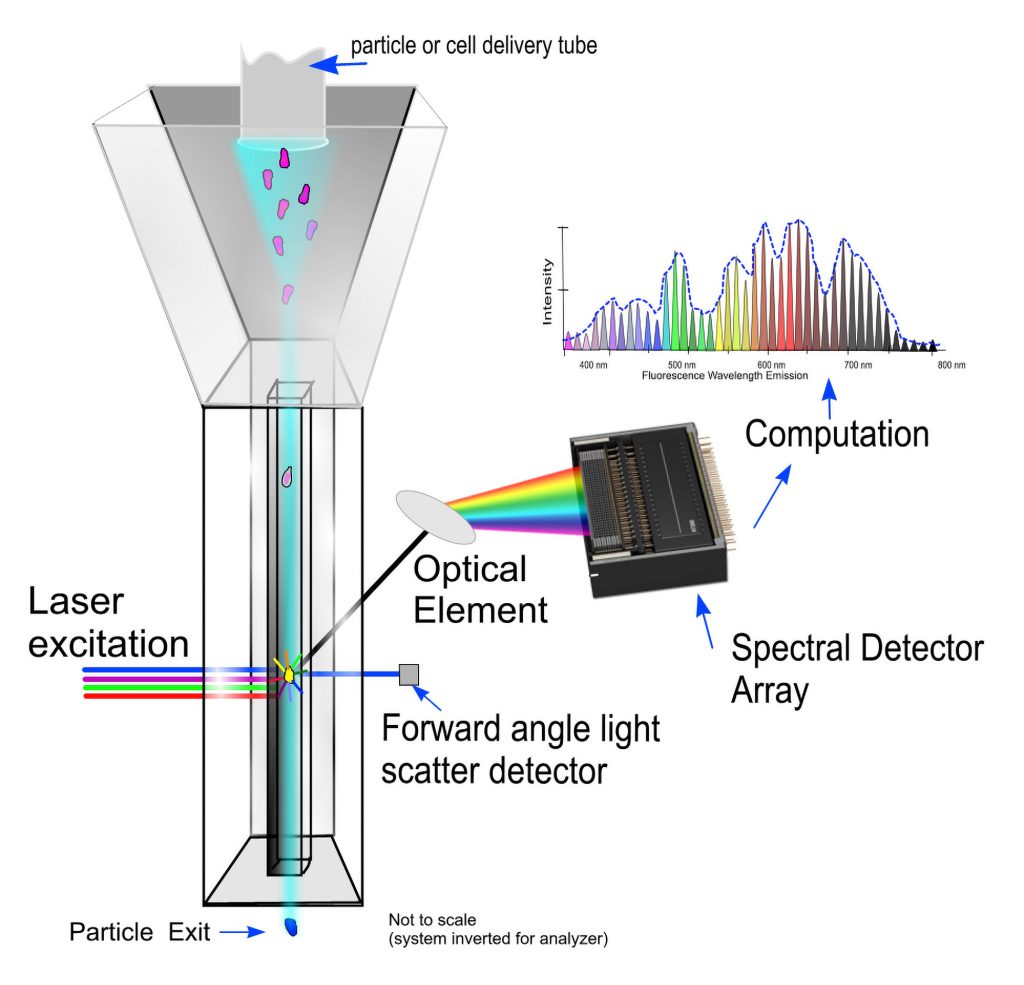
Unlike polychromatic flow cytometry, spectral flow cytometry does not require bandpass filters. For spectral flow cytometry, this is a significant advantage. Bandpass filters can miss a significant amount of signal, especially in multiparametric assays.
Robinson, who is distinguished professor of cytometry and professor of biomedical engineering at Purdue, invented the spectral cytometry technology in 2003 (and patented it in 2004). “Everybody was interested in this concept, and I gave talks all over the world,” he recalls, “but nobody wanted to do it.”
Like most novel technologies, spectral was slow to gain acceptance. Spectral wasn’t embraced straightaway because it lacked certain real-time capabilities. For example, as Robinson notes, spectral lacked the computing capacity to perform real-time spectral unmixing. Nonetheless, Robinson’s spectral technology was eventually licensed by Sony Life Sciences (in 2012) and by Thermo Fisher Scientific (in 2020).
The same year Sony licensed the technology, the company launched the first commercial spectral flow cell analyzer. The current version of the Sony instrument, the ID7000™ Spectral Cell Analyzer, can be configured with up to 7 lasers and up to 186 detectors, and it allows experiments with 44 colors or more. Robinson notes that until 2016, the maximum number of parameters in a flow cytometry had been stuck at around 28 to 30. He adds that in the years since, the number of parameters “jumped 30% because of spectral.”
“Expert systems and neural networks have an impact,” Robinson says of the willingness to embrace new trends in flow cytometry. If more powerful computer processing technology had been available sooner, he speculates, spectral cytometry might have penetrated the research world more quickly.
Artificial intelligence and machine learning
Machine learning, artificial intelligence systems, and neural networks are all being added to existing flow cytometry instruments such as the ones utilized by Ziv Porat, PhD, head of the flow cytometry unit at the Weismann Institute of Science in Rehovot, Israel. “It is actually one of the largest flow cytometry units in the world in terms of size and staff,” Porat details. It contains nine sorters, five analyzers, and a mass spectrometer; it supports graduate studies and research projects across five departments; and it serves over 400 users annually. “We also have two instruments for imaging flow cytometry,” Porat continues, “which is my specialty.”
He has the use of two ImageStream® imaging flow cytometers. The current versions of these instruments, which are available from the Amnis division of Luminex, can read up to 5,000 cells per second with real-time intensity compensation. They offer brightfield and darkfield capabilities and up to 10 fluorescence channels.
Porat, who has published 80-plus papers on imaging flow cytometry, and may hold a record in the field, has been working with these analyzers for over 11 years. He notes that an imaging flow cytometer is built much like any other flow cytometer. For example, cells pass through an imaging flow cytometer the same way they’d pass through a traditional flow cytometer. He adds, however, that an imaging flow cytometer detects cells differently: “Instead of a photomultiplier tube, we have a charge-coupled device camera, which can image at high speed.”
The advantages offered by imaging flow cytometry include “high throughput, uniform acquisition, multiple channels, and rare cell detection,” Porat remarks. Another advantage is the ability to detect 10 colors. This alone sets imaging flow cytometry apart from traditional microscopy, where only three to five colors can be detected.
“[Let’s say] you have a complicated population that has more than three to five markers, specifically immune cells,” Porat suggests. “Imaging flow cytometry can identify them.” Variations in image illumination seen with traditional microscopy are also reduced in flow imaging, he says, “because each cell passes separately and gets the same illumination as they pass the beam.” A disadvantage of flow imaging is its inability to take a Z-stack. (Each cell is imaged once and sent to waste.) Another disadvantage is slower flow processing. (Speed is constrained by the imager.)
In the past few years, Amnis has released two expert system software modules—a machine learning module and an artificial intelligence module. Both modules enhance imaging applications.
“The machine learning module allows you to combine many combinations of masks and features to create a super feature called a classifier,” Porat explains. This feature allows users to identify cells in a complex population more efficiently. Users needn’t create image masks to perform calculations based on size, composition, fluorescence distribution, and other features within whole cells or nuclei or other organelles.
The artificial intelligence module “is based on the image itself,” Porat says. “It has a neural network. It can cluster the images into different groups without the calculation of masks or features. And it can then classify them.” From there, users can select populations to train the system.
Precision flow cytometry detection methods
EV detection
Bruno Costa-Silva, PhD, a group leader at the Champalimaud Foundation in Lisbon, Portugal, studies extracellular vesicles (EVs) as biomarkers of cancer metastasis. “EVs mediate communication between cells locally and systemically, between tissues and organs,” Costa-Silva says. “EVs can do so because they carry all basic biomolecules, including proteins, DNA, nucleic acids, and lipids.”
In 2015, just before Costa-Silva left Weill Cornell Medical College to join the Champalimaud Foundation, he participated in research that was published in Nature Cell Biology, in a paper titled, “Pancreatic cancer exosomes initiate pre-metastatic niche formation in the liver.” Costa-Silva indicates that in this paper, the authors reported that “EVs are very proficient at going to future sites of metastasis and preparing these sites to receive metastasis.”
“In preclinical settings,” he continues, “people know that if you treat mice with inhibitors that block vesicle release, the inhibitors improve the animals’ response to immunotherapy and chemotherapy.” Detecting EVs in cancer patients for prognostic purposes became Costa-Silva’s goal at his new laboratory.
Costa-Silva has developed a flow cytometry technique that may revolutionize EV detection. The technique, which uses fluorescent markers and carboxyfluorescein diacetate succinimidyl ester dye, leads to considerable time savings. It can accomplish in hours tasks that can take days with traditional EV analysis methods.
“With traditional methods, you may be able to get one or two measurements from one tube of blood,” Costa-Silva elaborates. “It takes a long time. Sometimes it can take a week.” Traditional methods typically include several cycles of centrifugation, including ultracentrifugation, to separate first by size, then by density, followed by overnight blocks for specific protein content analysis. “We were looking for a method that could accelerate this process and then optimize the use of the sample,” he states. “If we could do 30, 100, or 200 tests, that would be amazing for the field.”
Costa-Silva notes that last September, some of his group’s most recent work appeared in Cells in a paper titled, “IgG+ Extracellular Vesicles Measure Therapeutic Response in Advanced Pancreatic Cancer.” He adds that his group managed to “detect a single protein from EVs in plasma linked with the chemotherapy response of pancreatic cancer patients.” Although he believes that this technology will be used for diagnosis and treatment monitoring, he admits that challenges still exist: “We do a good job of labeling one protein, but it is more difficult in some cases to label more than one protein of interest.”
Also, due to the small size of the particles examined, analyses can be complicated by artifacts such as antibody agglutinations, unless instruments are used that can distinguish between small particles. The instrument that Costa-Silva uses—the Micro-PLUS analyzer from ApogeeFlow—can discriminate between particles as small as 100 nm. “In our experimental setting, most extracellular vesicles are about 100–150 nm,” he says, “Bottom line, I don’t think size is a problem anymore if you have the right equipment.”
Variant DNA detection
Another researcher interested in applying flow cytometry at the subcellular level is Lei Chen, PhD, co-founder and chief technology officer of Rarity Bioscience. Chen co-founded Rarity in 2021 to commercialize the technology that had been developed in a laboratory led by Ulf Landegren, PhD, professor of molecular medicine at Uppsala University, Sweden.
“I invented the padlock-superRCA technology together with Ulf,” Chen recalls. This technology combines traditional flow cytometry and rolling circle amplification (RCA). It can be used to detect rare DNA variants, such as cancer mutations, in blood, bone marrow, and tissue samples.
The superRCA technique was described by Chen, Landegren, and other researchers last July in Nature Communications, in a paper titled, “Ultra-sensitive monitoring of leukemia patients using superRCA mutation detection assays.” The paper indicated that circulating tumor-specific somatic mutations could be useful for personalized approaches to therapy monitoring. In addition, the paper explained that with superRCA, mutant sequences are amplified via PCR and converted into single-stranded DNA circles.
Oligonucleotides template the reactions and serve as primers for RCA reactions. RCA products are ligated with padlock probes (specific for mutant or wild-type sequences) to form secondary RCA products. “The assay overcame the ligase fidelity issues by genotyping amplified target sequences in clusters,” Chen says. These clusters of single-stranded DNA objects, called superRCA products, form from each starting DNA circle.
“With the superRCA structure, all the secondary RCA products are held together,” Chen continues. The size of the superRNA structure allows for the direct detection of DNA variants within a biological fluid by flow cytometry. For the practitioner, using superRNA assays is similar to following traditional cell analysis protocols, Chen suggests.
“Since superRCA assays represent a targeting approach,” Chen says, “their greatest value is in analyzing known mutation targets at ultrasensitive performance.” In the Nature Communications paper, the researchers observed 12 acute myeloid leukemia mutations including mutations to IDH. An upcoming study of colorectal cancer will report that 42 mutations were detectable on a single portion of DNA with high sensitivity.
Chen asserts that superRCA assays may aid personalized medical approaches “by monitoring the relapse of the patient.” He adds that “lung cancer, colorectal cancer, and leukemia mutations are ready for service analysis.”
Panels for clinical trials
Sample recovery problems and collection volume problems beset the development of clinical trial assays for scarce cellular populations. “Both of these factors can be limiting,” explains Ben Fancke, PhD, interim associate director of operations, Pharma Services, NeoGenomics. “Flow is not only a critical arm in the diagnosis/prognosis of cancers, but a key platform for illuminating immune responsiveness to treatment.”
“We validate and perform oncology clinical testing in support of global clinical trials,” Fancke states. Representing NeoGenomics at the December 2021 Flow Cytometry Congress, Fanke discussed the company’s progress in developing a panel for the detection of scarce cell subtypes, specifically, subsets of myeloid-derived suppressor cells (MDSCs) and dendritic cells (DCs).
“MDSC/DC subsets are not ‘new,’ per se,” Fancke says, “but much of what is known comes from animal models rather than human research.” Both their scarcity and instability ex vivo has led to challenges in assessing these population in standardized clinical trials. “We hope that by creating flow panels like this one, we can open the possibility for clinical trials to adopt these for experimental testing,” he relates. “We also hope that these panels will, in turn, illuminate the influence of these populations during cancer associated treatments.”
“With rare cell subsets it is imperative to extract as much information from as small a sample as possible,” he points out. He anticipates that advances in flow cytometric technologies will “allow greater levels of detail to be extracted from each patient sample.”
"flow" - Google News
October 06, 2022 at 01:57PM
https://ift.tt/fvgRA4k
Flow Cytometry Rides Successive Waves of Innovation - Genetic Engineering & Biotechnology News
"flow" - Google News
https://ift.tt/17s349V
https://ift.tt/Qg5jIAe
Bagikan Berita Ini
0 Response to "Flow Cytometry Rides Successive Waves of Innovation - Genetic Engineering & Biotechnology News"
Post a Comment